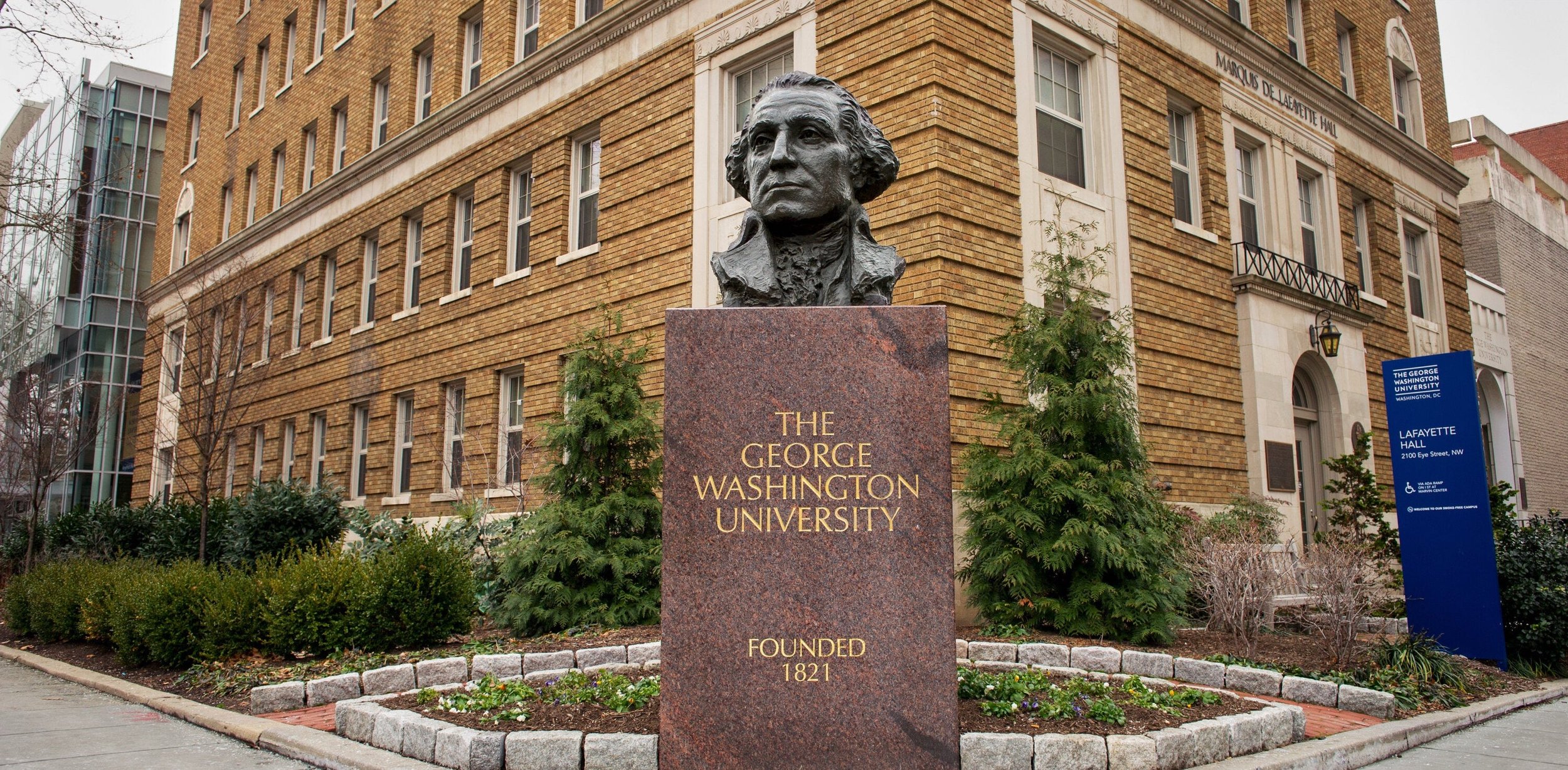
Susan Ariel Aaronson, Co-PI
Research Professor of International Affairs
George Washington University
Area of Expertise: Data and AI Governance
Susan Ariel Aaronson is a research professor of international affairs at GW where she is also the co-founder and director of the Digital Trade and Data Governance Hub. As co-PI of TRAILS, Aaronson directs research focused on data and AI governance. Her recent papers are on public participation projects in government AI strategies and data governance related to generative AI.
-
Susan Ariel Aaronson, Data Dystopia: The Inadequacies of Data Governance for Large Learning Models. Forthcoming: Centre for International Governance Innovation.
This article focuses on the data supply chain—the data collected and then utilized to train large language models and the challenges this supply chain presents to policymakers: The first issue focuses on how web scraping may affect individuals and firms which hold copyrights. The second issue focuses on how web scraping may affect individuals and groups who are supposed to be protected under privacy and personal data protection laws. The third issue is relatively new—it relates to the lack of protections for content and content providers that utilize open access web sites. The fourth issue stems from the lack of clear rules to ensure the veracity and completeness of datasets.
This report does not utilize a traditional methodology. Instead of looking at a representative sample of countries, I focus only on those governments have taken specific steps (actions, policies, new regulations etc.) to address LLMs or generative AI. I use these examples to show that policymakers do not seem to be looking at these modelsor the data supply chain in a systemic manner. A systemic approach has two components: First it recognizes that there are many different types of AI systems. Each of these systems has a large supply chain with different sources of data, that are linked to other systems designed, developed, owned, and controlled by different people and organizations. Data and algorithm production, deployment and use are distributed among a wide range of actors who together produce the system’s outcomes and functionality However, “these dynamics on interdependence, perpetual change, integration and consolidation produce supply chain in which responsibility for algorithmic systems is distributed between interdependent actors and visibility across the actors involved is low.”(Cobbe et al: 2023). Consequently, these systems are difficult to govern.
Secondly, variants of AI, including generative AI “involve a complex mix of applications, risks, benefits, uncertainties, stakeholders, and public concerns.” Indeed, no single entity is capable of fully governing them. Instead, as a report for the US National Academy of Sciences notes, policymakers must create “a governance ecosystem that cuts across sectors and disciplinary silos and solicits and addresses the concerns of many stakeholders.”[1] This assessment is particularly true for LLMs—a truly global product with a global supply chain with numerous interdependencies among those who supply data, those who control data, and those who are data subjects or content creators (Cobbe et al: 2023).
-
Zable, A., & Aaronson, S. A. (2022). Missing Persons: The Case of National AI Strategies, Centre for International Governance Innovation.
Governance requires trust. If policymakers inform, consult, and involve citizens in decisions, policymakers are likely to build trust in their efforts. Public participation is particularly important as policymakers seek to govern data-driven technologies such as artificial intelligence. Although many users rely on artificial intelligence systems, they don’t understand how these systems use their data to make predictions and recommendations that can affect their daily lives. Over time, if they see their data being misused, users may learn to distrust both the system and how policymakers regulate them. Hence, it seems logical that policymakers would make an extra effort to inform and consult their citizens about how to govern AI systems.
Herein we examined if officials informed and consulted their citizens as they developed a key aspect of AI policy, national AI strategies. According to the OECD, such strategies articulate how the government sees the role of AI in the country and its contribution to the country’s social and economic development. They also set priorities for public investment in AI and delineate research and innovation priorities. Most high-middle-income and high-income nations have drafted such strategies. Building on a data set of 68 countries and the EU, we used qualitative methods to examine whether, how and when governments engaged with their citizens on their AI strategies and whether they were responsive to public comment.
We did not find a model of responsive democratic decision-making, where policymakers invited public comment, reviewed these comments, and made changes in a collaborative and responsive manner. As of October 2022, some 43 of our 68 nations and EU sample had an AI strategy, but only 18 nations attempted to engage their citizens in the strategy’s development. Moreover, only 13 of these nations issued an open invitation for public comment and only four of these 13 provided evidence that public inputs helped shape the final text. Few governments made efforts to encourage their citizens to provide such feedback. As a result, in many nations, policymakers received relatively few comments. The individuals who did comment were generally knowledgeable about AI, while the general public barely participated. Hence, policymakers are missing an opportunity to build trust in AI by not using this process to involve a broader cross-section of their constituents.
-
Susan Ariel Aaronson, 2022. "Could a Global Wicked Problems Agency Inspire Greater Data Sharing?” CIGI Paper 273. CIGI Papers Series.
Global public goods are goods and services with benefits and costs that potentially extend to all countries, people, and generations. Global data sharing can also help solve what scholars call wicked problems-problems so complex that they require innovative, cost effective and global mitigating strategies. Wicked problems are problems that no one knows how to solve without creating further problems. Hence, policymakers must find ways to encourage greater data sharing among entities that hold large troves of various types of data, while protecting that data from theft, manipulation etc. Many factors impede global data sharing for public good purposes; this analysis focuses on two.
First, policymakers generally don't think about data as a global public good; they view data as a commercial asset that they should nurture and control. While they may understand that data can serve the public interest, they are more concerned with using data to serve their country's economic interest. Secondly, many leaders of civil society and business see the data they have collected as proprietary data. So far many leaders of private entities with troves of data are not convinced that their organization will benefit from such sharing. At the same time, companies voluntarily share some data for social good purposes. However, data cannot meet its public good purpose if data is not shared among societal entities. Moreover, if data as a sovereign asset, policymakers are unlikely to encourage data sharing across borders oriented towards addressing shared problems. Consequently, society will be less able to use data as both a commercial asset and as a resource to enhance human welfare.
This paper discusses why the world has made so little progress encouraging a vision of data as a global public good. As UNCTAD noted, data generated in one country can also provide social value in other countries, which would call for sharing of data at the international level through a set of shared and accountable rules (UNCTAD: 2021). Moreover, the world is drowning in data, yet much of that data remains hidden and underutilized. But guilt is a great motivator. The author suggests a new agency, the Wicked Problems Agency, to act as a counterweight to that opacity and to create a demand and a market for data sharing in the public good.
-
Aaronson, S. A. (2023). Building Trust in AI: A Landscape Analysis of Government AI Programs (CIGI Paper No. 272). CIGI Papers Series.
The Organisation for Economic Co-operation and Development’s (OECD’s) website on artificial intelligence (AI) policy (the OECD.AI Policy Observatory) is the world’s best source for information on public policies dedicated to AI, trustworthy AI and international efforts to advance cooperation in AI. Using the site as a source, the author sought to answer four questions:
→ What are governments doing to advance AI and trustworthy AI in their respective countries and internationally?
→ Do these governments evaluate or report on what they are doing?
→ Were the evaluations useful, credible and independent?
→ Did these evaluations inform best practice and trustworthy AI at the OECD?
The author’s review of the information contained on the site reveals that governments have yet to effectively evaluate their efforts. The 62 nations that reported to the OECD as of August 2022 generally reported initiatives designed to build domestic AI capacity and a supportive governance context for AI. This is understandable, as policy makers must show their constituents that they will deliver programs designed to meet their needs. Yet the author found few of these initiatives were evaluated or reported on. Consequently, policy makers were not effectively learning from their programs, as the author found only four out of 814 programs or 0.49 percent were evaluated.
In reviewing an early iteration of this paper, the OECD noted that most of the national AI initiatives were launched in 2019 and 2020, and it may be too early to effectively evaluate them. OECD staff also stressed that they encourage countries to evaluate their own initiatives. Finally, OECD commentors stated that they recommend that these governments think about which data they should gather to evaluate these programs in the future. But some of the programs funded by governments started decades ago. These governments have had years to develop a methodology to assess long-standing problems that is useful, credible and independent. Why have they not made such evaluations a priority?
The research also uncovered gaps between what governments said they were doing on the OECD website and what was reported on national websites. In some cases, the author did not find evidence of governmental action (for example, public consultations). In other cases, the links provided by governments to the OECD did not work. In addition, the author was surprised to find that only a small percentage of initiatives listed by governments included the keywords “trustworthy/trust,” “responsible,” “inclusive” or “ethical” in their titles, which may indicate that few initiatives pertained directly to building trust in AI or building trustworthy AI globally. The author’s research also found relatively few efforts to build international cooperation on AI, or to help other nations build capacity in AI.
In actuality, no one knows how to build trust in AI or whether efforts to promote trustworthy AI will be effective. Ultimately, this responsibility falls on the developers and deployers of AI and the policy makers who govern AI. But more understanding is needed to sustain trust in AI. Hence, nations that conduct evaluations of AI efforts are likely to build trust in both AI and AI governance. These nations are signaling that policy makers are competent and accountable and care about their fellow citizens who rely on AI.
-
Aaronson, S. A. (2022). A Future Built on Data: Data Strategies, Competitive Advantage and Trust (CIGI Paper No. 266). CIGI Papers Series.
Abstract: In the twenty-first century, data became the subject of national strategy. This paper examines these visions and strategies to better understand what policy makers hope to achieve. Policy makers in many countries have long drafted strategies for economic growth or to govern various technologies. Some of these strategies may be designed to achieve comparative or competitive advantage. But data is different from other inputs: it is plentiful, easy to use and can be utilized and shared by many different people without being used up. Moreover, data can be simultaneously a commercial asset and a public good. Various types of data can be analyzed to create new products and services or to mitigate complex “wicked” problems that transcend generations and nations (a public good function). However, an economy built on data analysis also brings problems — firms and governments can manipulate or misuse personal data, and in so doing undermine human autonomy and human rights. Given the complicated nature of data and its various types (for example, personal, proprietary, public, and so on), a growing number of governments have decided to outline how they see data’s role in the economy and polity. The author based this study on a sample of 51 nations plus the European Union from various regions, income levels and digital prowess. There is a correlation between income, democracy, levels of digital prowess and data governance. Approximately one-fifth, or 10 governments, issued national data strategies, delineating how various types of data could contribute to their nation’s social and economic development. All of these nations are characterized as high income by the World Bank, except for China, which is an upper middle-income country. Two are authoritarian. All have high levels of digital prowess. Despite these differences, all of the plans aim to expand the scale and variety of data, increase skill endowments, build data infrastructure, and use governance (encourage network effects, expand free flow of data, and so on) to enhance the digital economy in their nation. Some of these plans make it quite clear that these nations hope to achieve competitive advantage in data-driven sectors. Very few policy makers see data as a public good. Sixty percent of these nations want to build comparative advantage in data-driven sectors, while 70 percent use these data governance strategies to build trust in their policies. While it is too early to evaluate the effectiveness of these strategies, policy makers increasingly recognize that if they want to build their country’s future on data, they must also focus on trust.
-
Aaronson, S. A. (2023, June 15). How to Regulate AI? Start With the Data. Barron's.
The article discusses the importance of data in the development and regulation of AI. Aaronson argues that while AI developers rely on large data sets to train their systems, U.S. policymakers do not view data governance as a significant means to regulate AI. The author suggests that data governance is an effective way to regulate AI, as demonstrated by the European Union and more than 30 other countries that provide their citizens with a right not to be subject to automated decision-making without explicit consent. The article also highlights the risks of relying on scraped data sets, which can contain incomplete or inaccurate data, leading to problems of bias, propaganda, and misinformation. Aaronson concludes by suggesting several steps for Congress to take in governing AI, including passing a national personal data protection law, requiring the Securities and Exchange Commission to develop rule-making related to the data underpinning AI, and re-examining the legality of web scraping. She focuses on the SEC, because these firms control ever more of the world’s data, and they should not be opaque about how they collect, utilize, and value data. In addition, the SEC requires firms to report on hacks of their data, hence they are already using corporate governance to regulate some aspects of data.